We’ve committed to achieve net zero by 2040
To get there, we’ve set near-term science-based targets to limit warming to 1.5° C.
Our highlights
We signed our first virtual power purchase agreement to match our in-office electricity and work-from-home energy use in the United States. We also launched our Don’t #@!% the Planet guide to how we’ve tackled climate action.
Where we fell short
Our travel emissions rebounded and are now higher than when we set our baseline – a risk we knew was coming as travel restrictions lifted.
Where we're going
Reducing emissions remains our top priority, and to get there, we’ll focus on building better leadership accountability and visibility into our emissions data so we can act faster in a changing business and regulatory environment.
We believe in the power of diversity
We aim for nothing short of equity for every Atlassian and are committed to an authentic culture of inclusion.
Our highlights
We conducted our first global pay equity audit, finding no observable statistically significant gaps in salary or equity on the basis of either gender globally or race/ethnicity in the U.S. We also assessed our talent programs and continued to iterate our approach to ensure equity for all Atlassians.
Where we fell short
The velocity toward greater representation for Atlassian women globally and Hispanic/Latinx Atlassians in the U.S. slowed this year. Despite our best efforts, this reinforces what we know to be true: Progress isn’t always linear for increasing the representation of all underrepresented groups.
Where we're going
We’ll continue embedding bias mitigation into our hiring and talent processes; enabling leaders to drive diversity, equity, and inclusion outcomes; amplifying voices of our employee resource groups; and measuring our outcomes.
Transparency and accountability live at the core of our business
These form the foundation of our human rights approach, as do our values, mission, and the UN Guiding Principles on Business and Human Rights.
Our highlights
Alongside the launch of Atlassian Intelligence, we shared Responsible Technology Principles, which identify five tenets that outline how we uphold our values when it comes to building, deploying, and using new technologies like artificial intelligence.
Where we fell short
We designed frameworks to conduct due diligence and improve our grievance mechanisms but have not yet implemented these, as we made trade-offs to focus on the explosion of generative AI.
Where we're going
Now that we’ve built human rights and ethical commitments and frameworks, we will work to further integrate these into our operations and make them usable for Atlassians in their day-to-day work, including due diligence, annual stakeholder engagement, and responsible technology programs.
We believe education and business have the power to change the world
We created the Atlassian Foundation with that belief.
Our highlights
The Atlassian Foundation donated US$11.5 million and 4,700 free or deeply discounted Community product licenses to nonprofits, and we surpassed 60,000 hours of employee volunteering during the year. We continued to partner with 16 education changemakers.
Where we fell short
We identified that a gap in product and practices support is affecting nonprofit teams, as many of these teams don’t have the same resources, capacity, and technical skills needed to effectively adopt and implement our products.
Where we're going
We’re refreshing our Atlassian Foundation strategy to scale up our operations, and we’re excited about making fewer, bolder moves in the next year and beyond to continue to back social impact teams with a combination of Atlassian’s funding, people, products, and practices.
Download our Sustainability Report
“Meaningful change can’t be done alone. We are urging companies of all shapes and sizes to join us in making this a year of saying yes to ESG.”
– Mike Cannon-Brookes and Scott Farquhar, Co-Founders and Co-CEOs
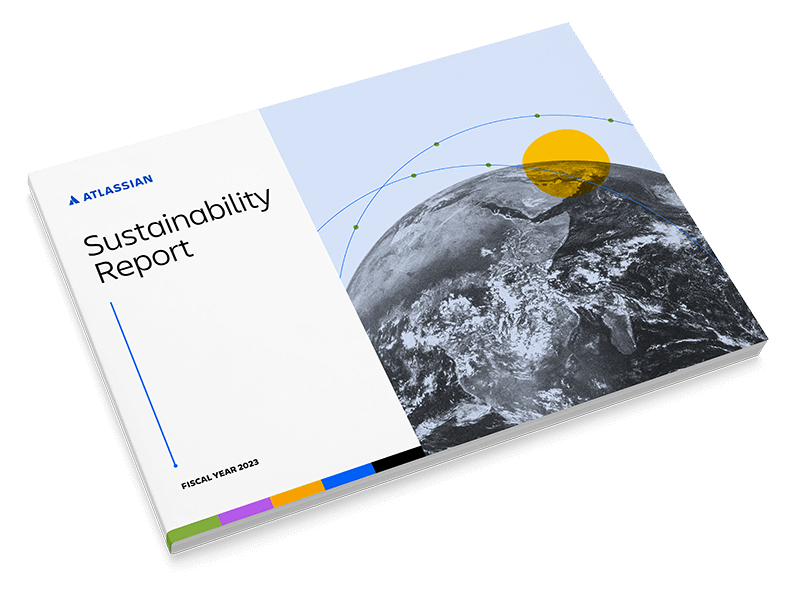
Want to see how we did in past years?
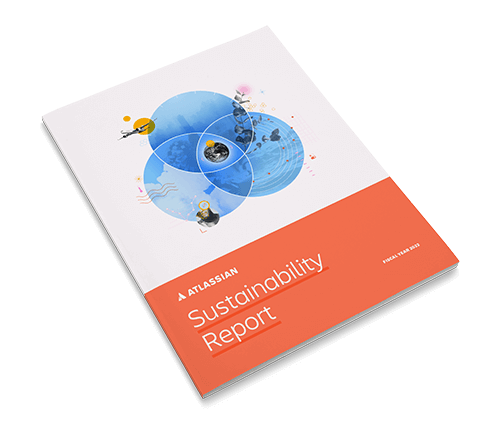
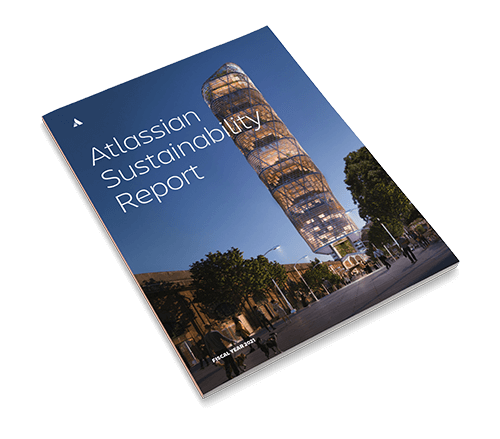
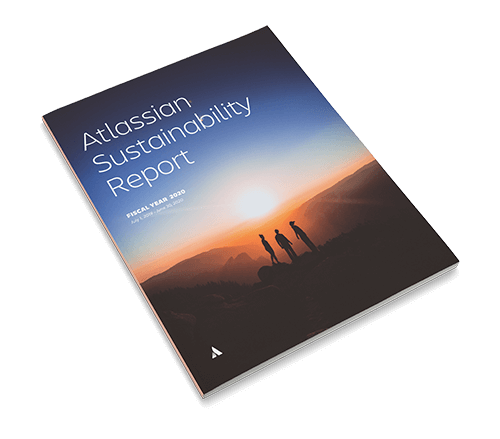
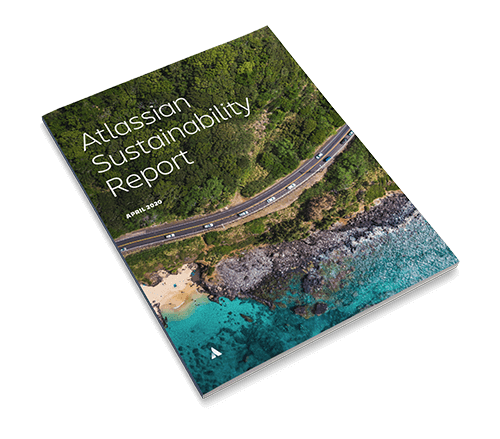