When teams are fully remote, it can be difficult to track down the right materials and the relevant colleague to move a project forward. Not only that, redundant tasks and clunky communication cycles slow down productivity. The ability of technology to solve these challenges has never been more evident than this year, as the majority of knowledge workers went remote overnight.
Atlassian is on a mission to help teams unleash their full potential through collaboration. Over the last few years, we’ve been building the Atlassian Platform that provides a common cloud infrastructure to create powerful connections across our products, making collaboration faster, more predictable, and secure. Using the Atlassian Platform, we have the ability to aggregate user patterns from over 150,000 customers of our cloud products to understand how work gets done and how teams interact at scale.
With these insights, we’ve leveraged machine learning to build predictive, smart experiences in our products to make teams more productive. Built on the Atlassian Platform, smarts is the subtle, often unnoticeable application of data-driven algorithms and machine learning techniques that help teams accomplish their tasks and speed up collaboration.
Today, we’re announcing general availability of smart capabilities for Atlassian cloud products to enable a more powerful and seamless collaboration experience. New features include smart search in Confluence Cloud, Jira and Jira Service Desk Cloud, and predictive pull request experience in Bitbucket Cloud. As these capabilities have been rolling out to customers over the last few months, we’ve scaled up to deliver millions of smart predictions each day to simplify teamwork. Coming soon, smarts will be able to cluster similar tickets in Jira Service Desk, making IT teams more efficient. Read below for all the details!
Find relevant information faster with predictive cross-product search
With the shift to remote work – and without the ability to tap our coworkers on the shoulder – finding the right document has never been more time-consuming. Leveraging machine learning, we’ve improved search across Confluence and Jira in the cloud to help you find the information you care about.
When you and a colleague on another team both search for “roadmap,” you’re likely each looking for different things, even though you’ve typed the same word. By identifying what you’ve recently worked on, smart search delivers a personalized search experience, sharing the most relevant document specifically for you.
We’ve taken search to the next level, optimizing it to show instant search results, based on what we think you’re going to look for, while we wait for smart search to pull through.
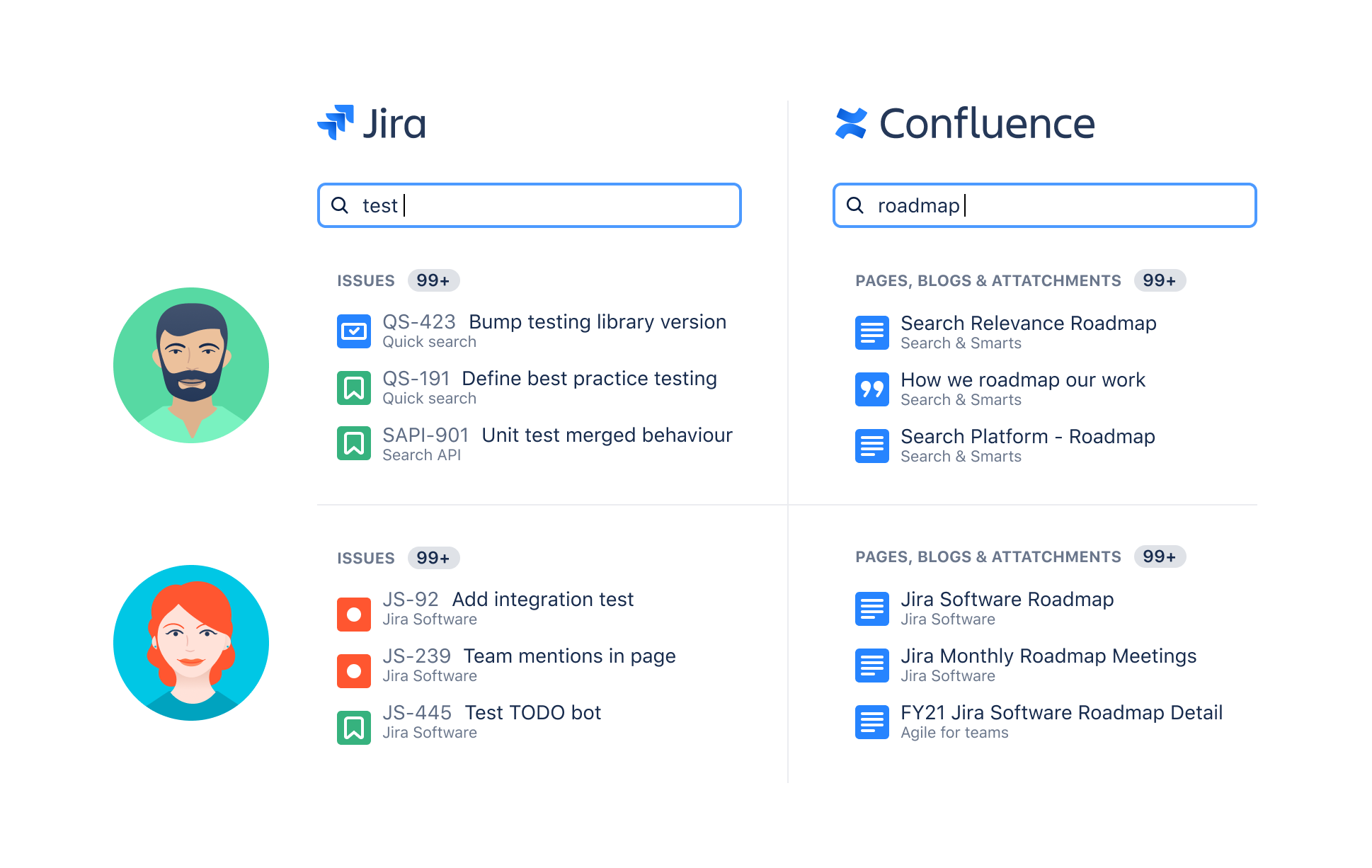
We’ve also added intelligent filter controls to the search experience in Jira and Confluence. They predict filters you’ll likely choose to narrow down the search scope by contributor and by spaces you work in. We’ve found that, when using filters, users select the intelligent suggestions 89 percent of the time.
But finding things isn’t just about running a search. We’ve used smarts to help kick-start your day at start.atlassian.com, which displays a personalized overview of Confluence documents you’ve worked on and other Atlassian products you’ve worked in. Today, 25 percent of customer clicks in Start are based on the intelligently suggested recommendations.
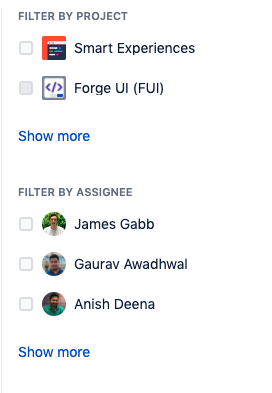
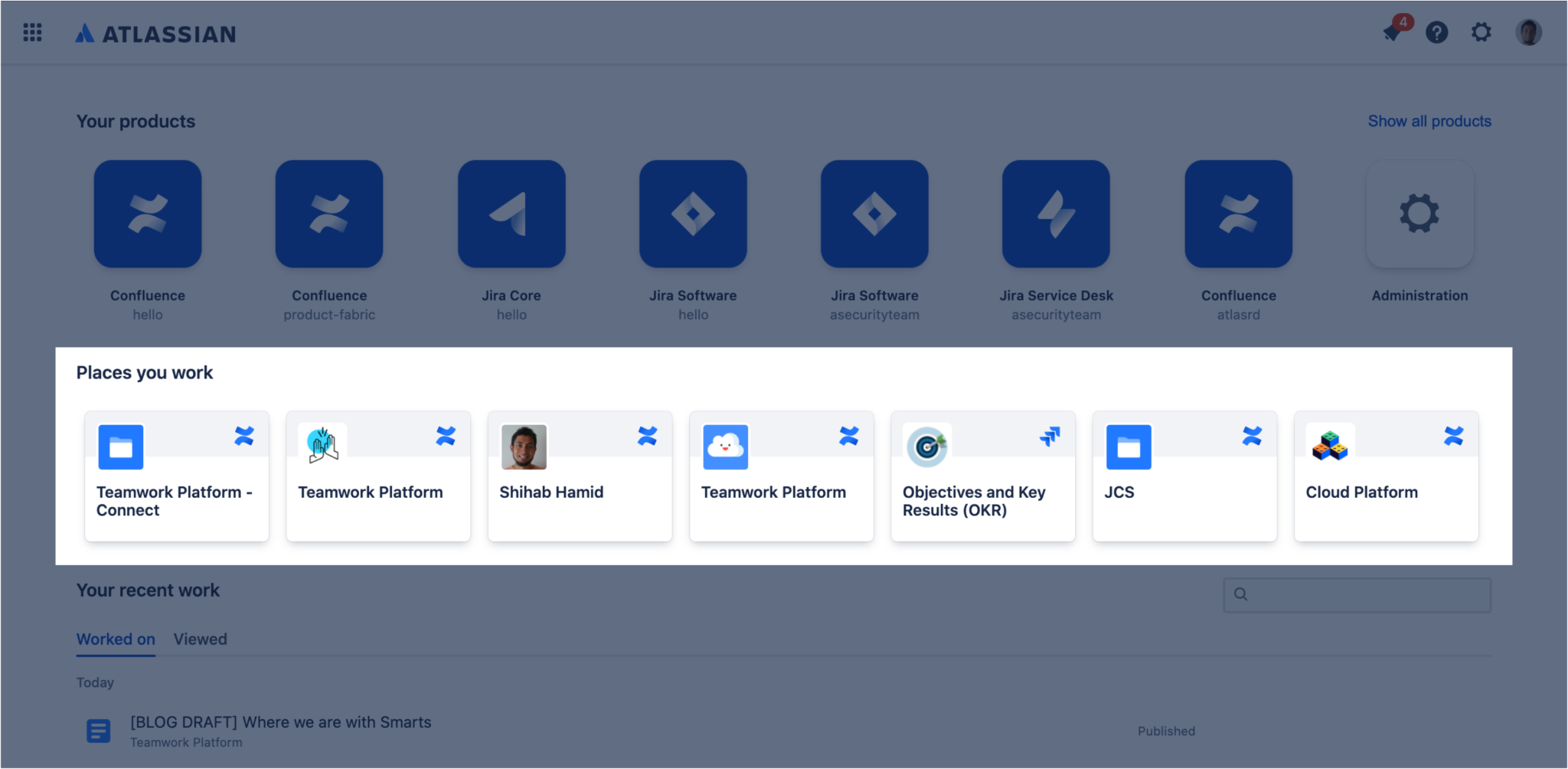
Accelerate collaboration by bringing in the right people
Collaboration is all about pulling in the right person at the right time to complete a project or move it forward. While this might not sound like a huge problem when you’re at a small startup of 10, as organizations grow, this seemingly simple experience can become frustrating. It’s suddenly harder to find the right person, and time spent tracking down individuals can add up throughout the day.
With predictive user mentions in Jira and Confluence, smarts recommends a list of people to bring into a project by knowing who you regularly work with and what you’re working on.
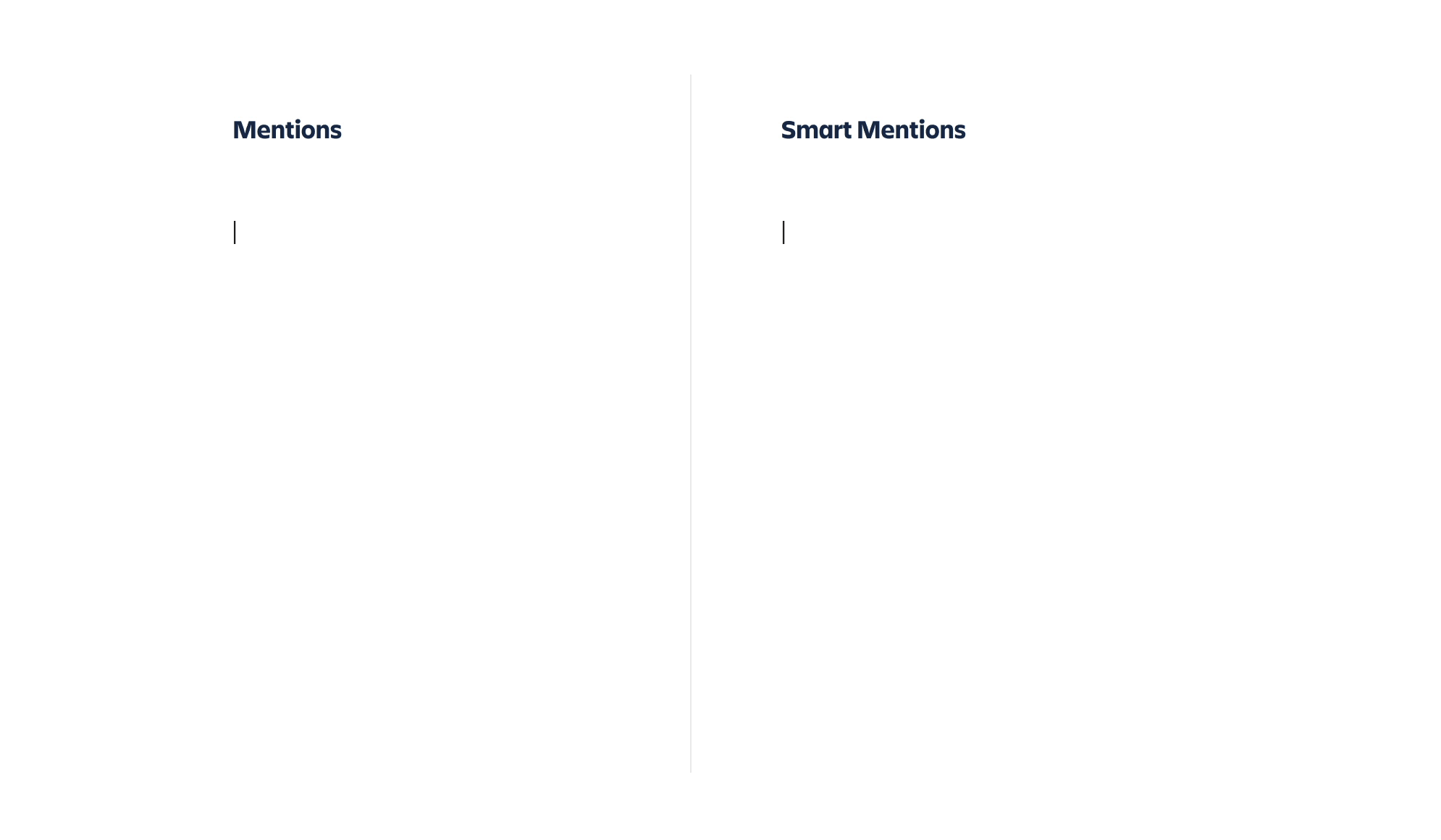
To take smarts even further, we’ve developed predictive user pickers. They suggest relevant teammates to collaborate with in different scenarios across our products, without needing to type a single character.
Here are some examples of how we’re making contextually relevant suggestions across our products:
Issue assignment in Jira: We know who’s active on the project, and we know who works on similar issues. By learning from past behavior, we can predict the top five most likely assignees with an accuracy of 86 percent.
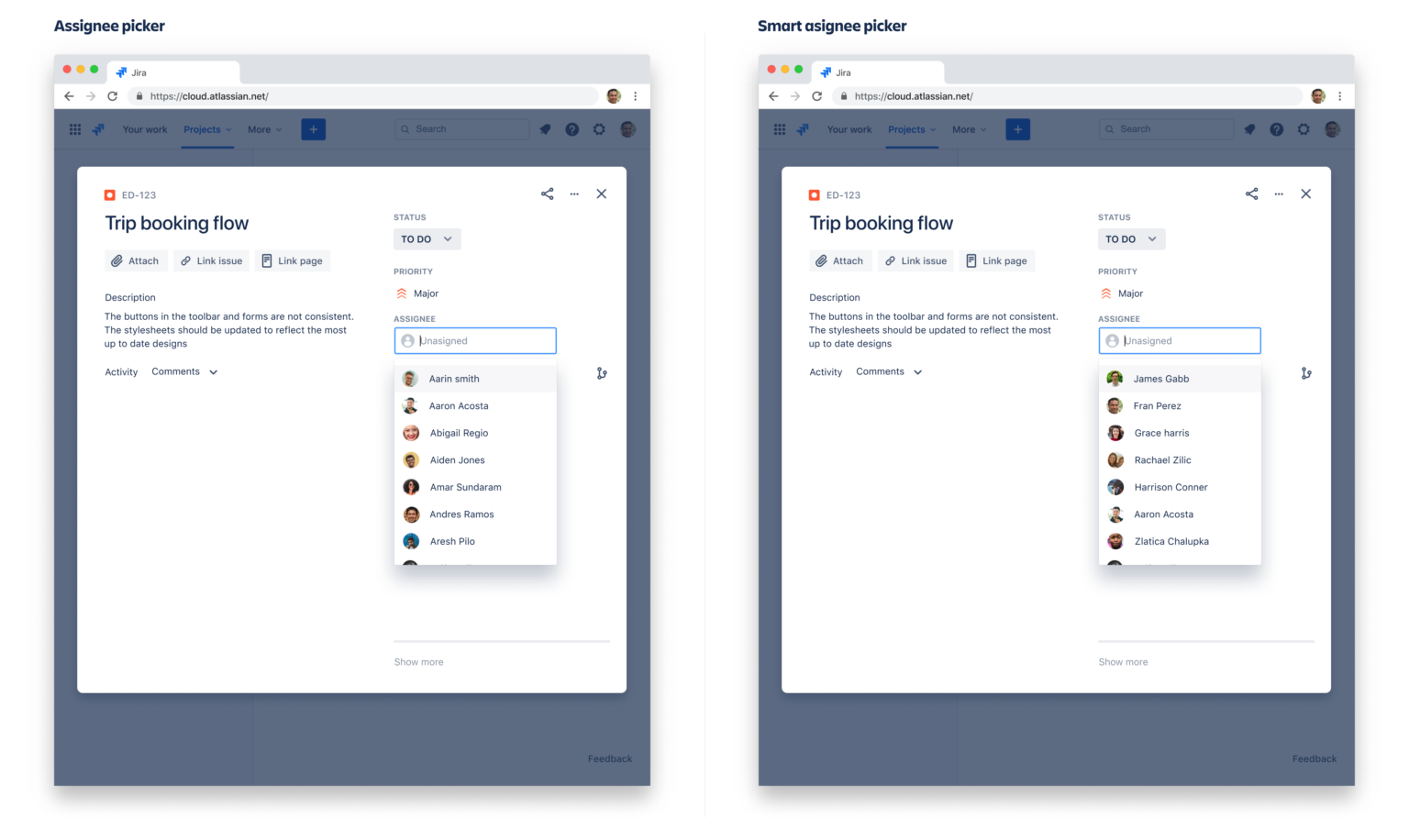
Page restrictions in Confluence: Smarts can identify who you collaborate with and what they typically work on, so we can predict who you may want to restrict from viewing a page.
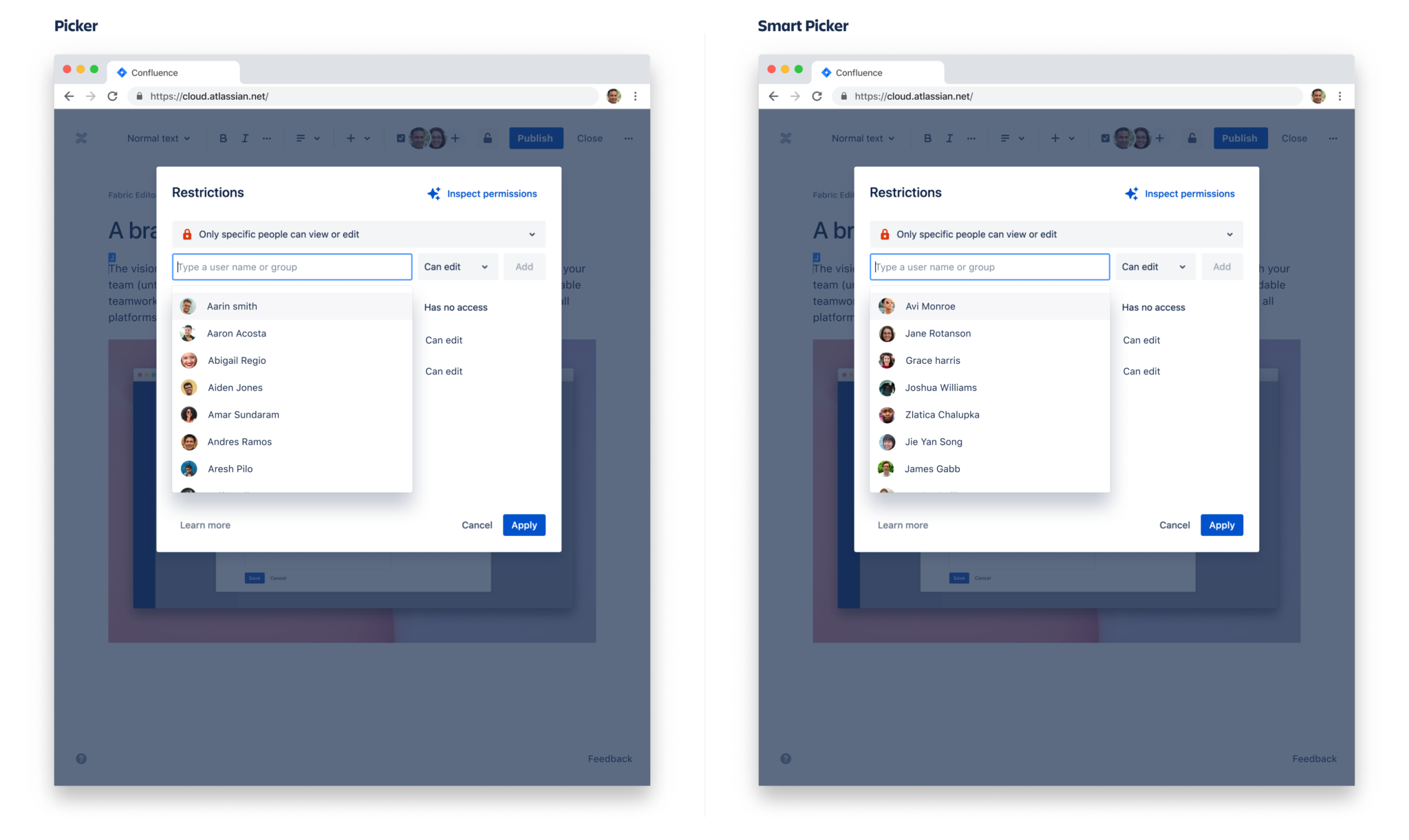
Pull request reviewers in Bitbucket: We know who works on a particular repository and has been pulled into similar pull requests in the past, so we can predict the best reviewers for a pull request.
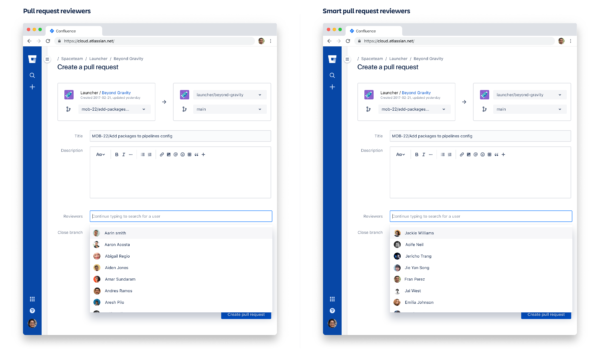
Speed up triage to resolve issues faster
If you work in an IT department, you likely spend a lot of your day combing through service desk tickets that address issues with common overlap. We’ve applied smarts in Jira to accelerate issue triage, so you can spend less time organizing and more time solving problems.
With hundreds of service desk tickets to get through each day, triaging similar tickets all at once can be a huge time saver for IT teams. Soon, we’re launching a new feature that will allow users to cluster similar tickets in Jira Service Desk.
This same predictive, natural language technology can be used in Jira to group together similar bug reports or features requests, linking incidents to tickets in Jira Service Desk and displaying related knowledge base articles in Confluence.
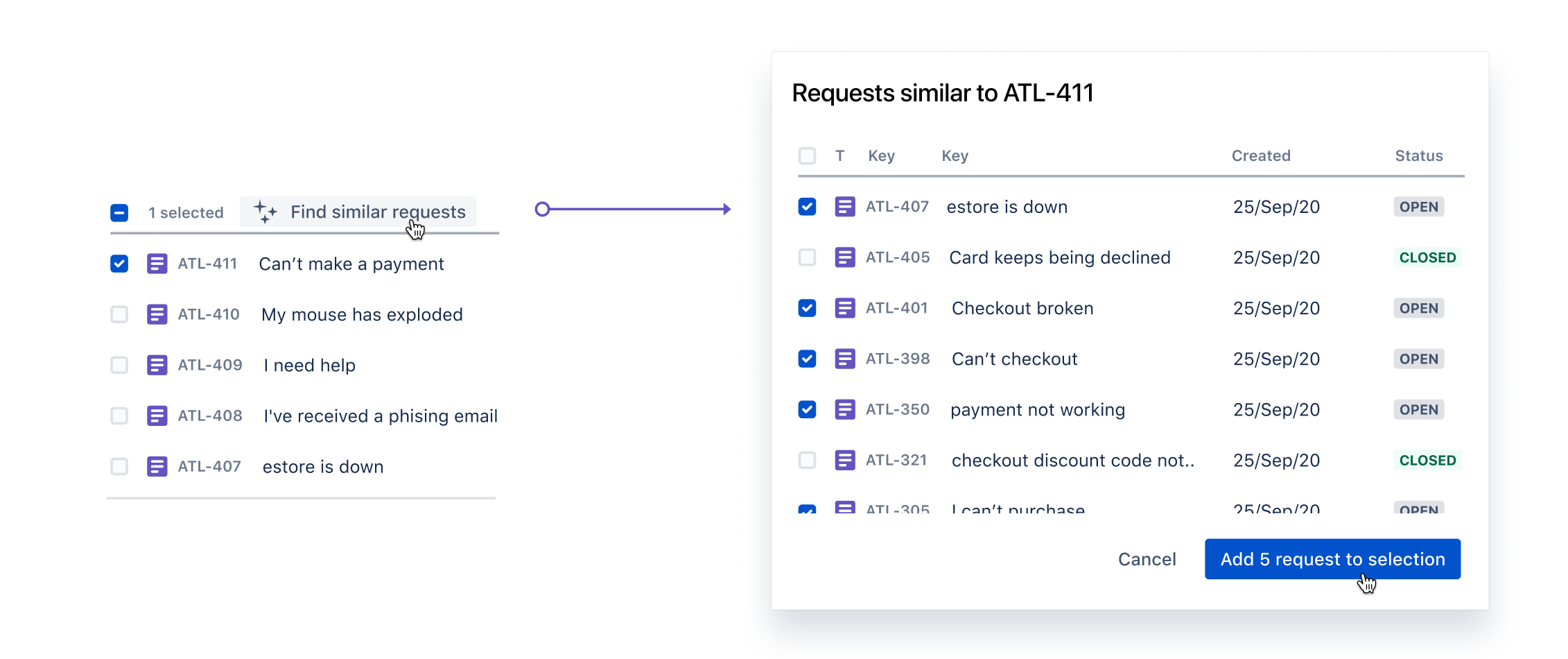
By learning from historical data, we’ve made many fields in Jira and Jira Service Desk intelligent. While you’re filling in components, labels, and versions of an issue, predictive fields surface the most relevant suggestions.
We can accurately predict the top five suggestions for common issue fields, including:
Predictive components: 79 percent accuracy
Predictive labels: 75 percent accuracy
Predictive versions: 75 percent accuracy
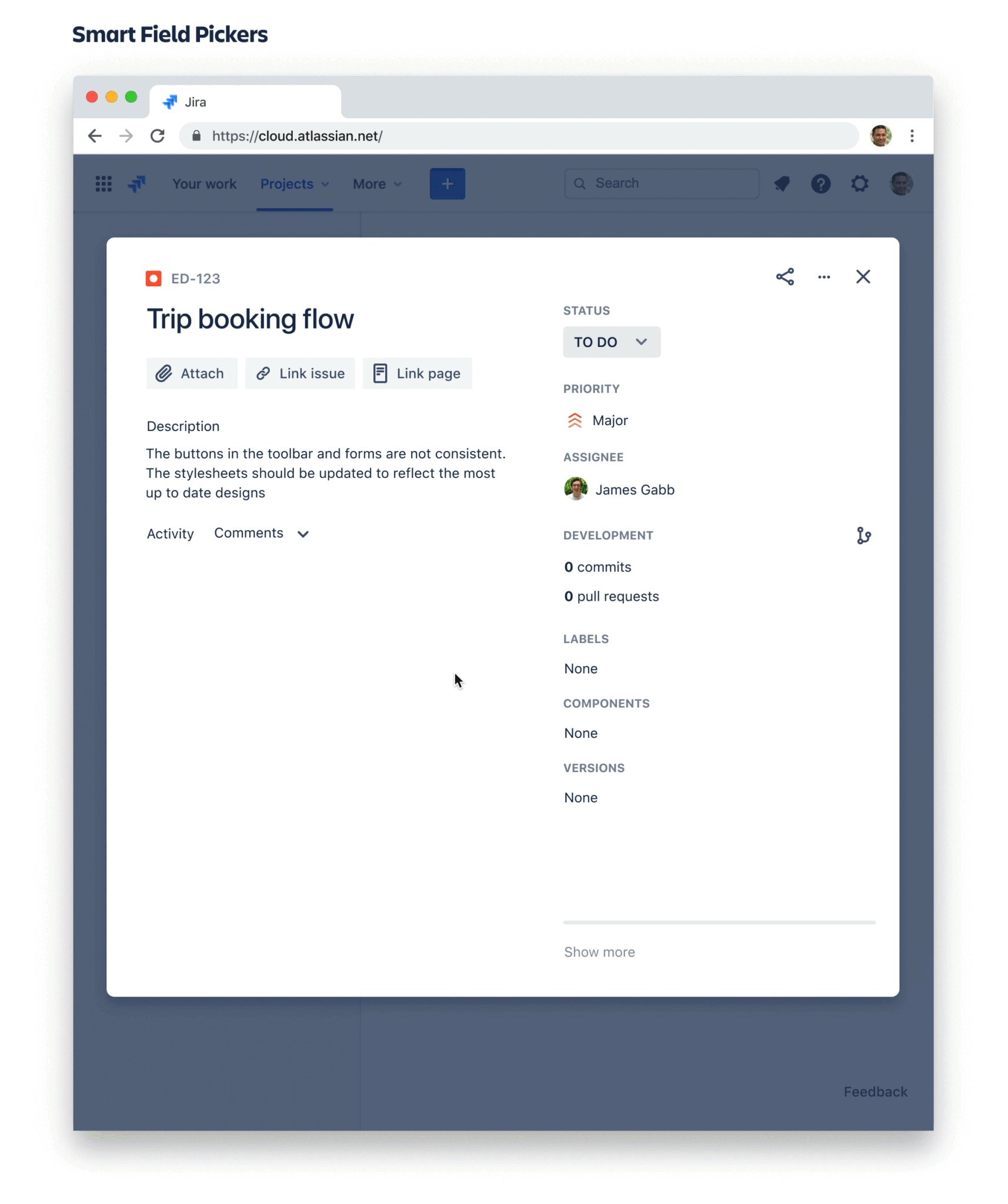
A smart shift forward
This is just the start of Atlassian’s work to incorporate smarts into our products and platform. We’re always looking for more ways to alleviate repetitive work so that teams can focus on their core mission. We’re building the smartest work collaboration platform on the market – because we’ve learned from how the best teams work.